Artificial Intelligence, agents, and prompting: how the new "smart electricity" will light up the future
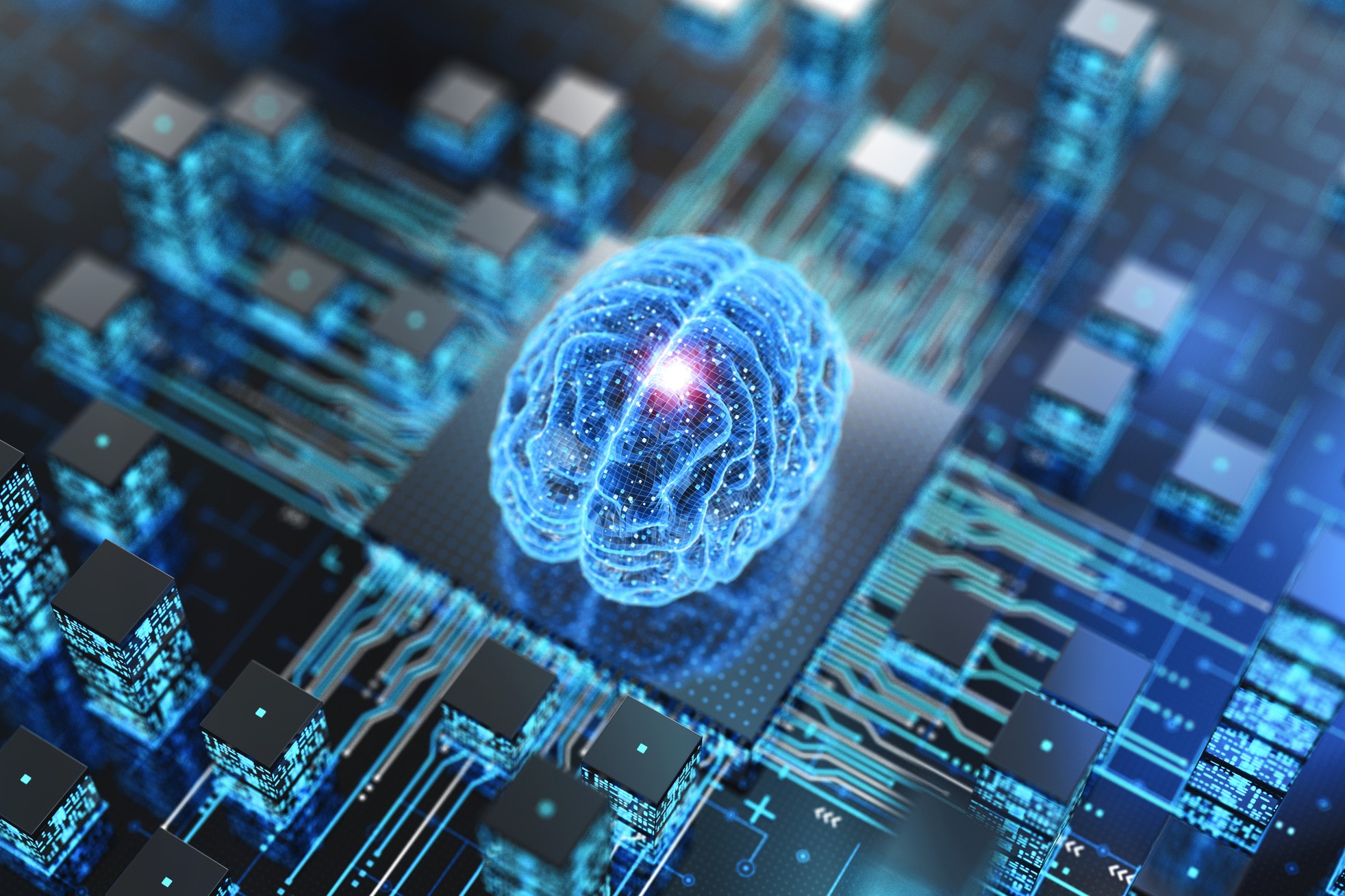
We are living in an extraordinary time, right in the midst of a technological revolution that is transforming the way we work and innovate. If - like me - you have experienced the Web 1.0, Web 2.0, and Web 3.0 eras, you will understand the pervasive impact that artificial intelligence will have on our future. Today, AI is much like electricity at the beginning of the 20th century: it is changing the world in ways we can only partially see. There will be companies and individuals who know how to fully leverage it - reinventing products, services, and jobs - and others who will struggle to adapt. The difference will largely depend on the attitude with which we face this revolution: fear or curiosity, resistance or continuous learning. History teaches us that technologies are not fate but tools - it is up to us to steer them toward the common good.
Artificial Intelligence (AI) is not just a technology but a true catalyst that pushes us to rethink how we think, decide, and act. Today, AI increasingly permeates both daily life and professional environments - from automatic suggestions on smartphones to strategic business analysis. In the face of this silent yet profound revolution, simply adopting new software is not enough - we need a shift in perspective. AI forces us to reconsider traditional decision-making and organizational processes, prompting us to challenge old habits and imagine new models of work and society. In this introduction, we will explore why AI should be understood as both a cultural and technological transformation and how it is becoming a driving force of innovation that touches the very core of our daily actions.
In other words, artificial intelligence represents a paradigm shift: from a passive tool to an active partner in our activities. This means that AI not only helps us do things faster but also changes the "how" and "why" behind our actions. A manager today, for instance, can use an AI agent to support strategic decisions, shifting their role from a mere decision-maker to the orchestrator of a hybrid human-machine team.
The Evolution of AI
To understand the current impact of AI, it is useful to briefly trace its historical evolution. The roots of artificial intelligence date back to the 1950s: in 1956, during a workshop held at Dartmouth College, the term "Artificial Intelligence" was officially coined. In that pioneering era, enthusiasm was sky-high - many believed that within a few decades, machines would match many human capabilities. Early programs aimed to imitate logical and mathematical reasoning, and in the 1960s and 1970s, the so-called expert systems emerged - software capable of making decisions in specific domains by following manually defined rules.
However, initial expectations were premature: limited computing power and the complexity of human "common sense" led to slower-than-expected progress. Periods of enthusiasm were followed by phases known as "AI winters," during which funding and interest declined due to the lack of tangible results.
The real breakthrough came between the late 20th and early 21st centuries. On one hand, the exponential increase in computing power - combined with the availability of vast amounts of digital data - paved the way for innovative methods. On the other hand, the scientific community made significant progress with artificial neural networks and machine learning algorithms, which could learn from data rather than just following static rules.
A symbolic moment came in 1997 when IBM's Deep Blue computer defeated world chess champion Garry Kasparov - proof that machines could surpass humans in highly complex yet well-defined tasks. From that moment on, milestones followed one after another: in 2012, a deep learning-based computer vision system surpassed all previous records in image recognition, effectively kickstarting the modern AI boom. In 2016, Google DeepMind’s AlphaGo program defeated the world champion of Go - a game long considered too sophisticated for a computer - demonstrating the power of advanced machine learning.
Generative AI and agents
We have now entered the era of generative AI and agents. Tools like OpenAI's GPT language models, Google's Gemini project, Anthropic's Claude, and Deepseek have revolutionized the landscape, enabling machines to generate original content—texts, images, code, and more. This new wave has brought AI into the spotlight like never before. Consider ChatGPT: launched at the end of 2022, it reached millions of users within weeks, introducing the general public to the potential (and limitations) of conversational AI.
Unlike early AI systems that were manually programmed, these modern models learn linguistic patterns from vast amounts of textual data, allowing them to answer questions, translate, summarize, and even engage in surprisingly natural conversations. The evolution of AI from academic laboratories to business offices is the result of decades of research, but the current boom is driven by a convergence of factors: low-cost computing power (cloud computing), massive online datasets, and algorithmic breakthroughs that enable the training of increasingly complex models.
We have transitioned from "artificial" AI, which merely imitated certain rules of intelligence, to "autonomous" AI, capable of learning patterns and creating. This evolution continues at an accelerating pace, laying the groundwork for the widespread adoption of AI in society and businesses.
A change in mindset
The effective adoption of artificial intelligence requires not only new technical skills but also (and above all) a shift in mindset. In many cases, the biggest obstacle is not the technology itself but the way people and organizations approach it. We are used to thinking about work and decision-making in a certain way, and AI challenges these habits, which have been ingrained over decades of practice. This requires a conceptual leap: from direct execution to intelligent coordination, from meticulous management to calibrated trust in machines, from instinct to data-driven analysis. In short, the transition involves:
- From “doing” to “making do”: Traditionally, the value of a professional or a company was also measured by their ability to directly perform certain tasks (writing reports, analyzing data, carrying out manual operations). With AI, many of these activities can be automated or performed more quickly by software systems. The new added value, therefore, lies in knowing how to make machines do what they do best. For example, instead of manually drafting a document, a manager can have AI generate an initial draft and then refine it. This does not diminish the human role but transforms it: the key skill becomes orchestrating work, effectively distributing tasks between people and algorithms.
- From control to trust: In traditional management, especially at the managerial level, there is often a tendency toward direct control over every detail - to ensure that everything is done "by the book." The introduction of AI, however, requires a greater degree of trust: one must learn to delegate certain decision-making or operational processes to machines and trust their results (while maintaining a level of supervision). This can be culturally challenging: it means accepting that an algorithm can make decisions (such as automatically selecting candidates in an initial recruiting phase or approving flagged financial transactions after an automated check) that a human would have made in the past. Trusting AI does not mean turning off critical thinking - on the contrary, it requires monitoring and validating results - but it does mean not obsessively intervening at every step and recognizing when the machine can perform a task well. Essentially, the manager becomes a guardian of AI: they train its initial criteria, check its outputs, but then allow it to operate autonomously within defined boundaries, intervening only when necessary.
- From intuition to analysis: Many business decisions and strategic choices, especially in the past, were based on previous experience and the intuition of decision-makers. The “instinct” of a leader or professional was seen as fundamental. With the advent of advanced AI systems, we now have access to large-scale data analysis and sophisticated predictive models. The new approach rewards the ability to interpret this data and trust analytical evidence, even when it contradicts initial assumptions. This does not mean devaluing human intuition—which remains valuable, particularly in big-picture thinking and creativity—but rather combining it with data-driven rigor. In other words, data-driven decision-making becomes the standard.
Adopting this new mindset is not immediate. It requires investment in training but also the promotion of an environment where mistakes are seen as learning opportunities. In a context where innovation means experimenting alongside machines, it is essential to accept that AI will sometimes make mistakes or propose counterintuitive solutions. The human role will then be to guide AI, correct its course when needed, and learn from the feedback the machine provides. Organizations that successfully embrace this shift in mindset often experience not only increased productivity but also greater job satisfaction: people are freed from tedious and repetitive tasks and can focus on higher-value activities, whether creative or strategic, feeling supported by AI in routine work.
Prompting as the new literacy
If AI is a powerful "engine," prompting is the new way to steer it using natural language. With generative AI (such as GPT models, advanced chatbots, etc.), the ability to communicate effectively with machines through prompts - textual instructions, questions, or inputs formulated correctly to achieve the desired outcome - has become essential. Knowing how to prompt an AI is now considered a new form of digital literacy, comparable in importance to using a search engine or writing basic code. In other words, just as learning to use a computer or navigate the internet became fundamental in recent decades, in the coming years, knowing how to interact with AI systems through well-structured requests will be just as crucial.
But what does prompting actually mean? It means learning to communicate with AI in a clear and effective way. A well-crafted prompt provides sufficient context and details to the AI model, guiding it toward the expected response or output. A good prompt should be clear, contextualized, and instructive.
In businesses, prompting is becoming an integral part of digital transformation: training employees to interact with AI enables them to fully leverage its capabilities. The difference between an inexperienced user and an expert in prompting can be substantial in terms of the quality of results obtained from AI. For this reason, courses and guides on prompt engineering are emerging, and some companies have begun incorporating this skill into their internal training programs. Knowing how to ask the right questions to a machine is becoming as important as knowing how to find the right answers for a human.
Ultimately, prompting is the key to unlocking the potential of the next generation of AI: without a well-posed question, even the most advanced AI will struggle to provide a useful answer.
AI and generational differences
The widespread introduction of artificial intelligence in the workforce and society is highlighting an important generational divide. As is often the case with technological innovations, different generations embrace and experience them differently. Digital natives - primarily the younger generations, such as Millennials and Gen Z - grew up surrounded by computers, the internet, and smart devices. For them, adapting to a new AI tool is often intuitive, if not even exciting. In contrast, those who were not born in the digital age (such as Generation X and Baby Boomers) had to learn to use technology in adulthood: for these people, embracing AI can represent a cultural challenge.
These differences manifest in various ways. Younger people tend to experiment with AI without hesitation: they try new apps, play with chatbots as if they were virtual companions, and spontaneously integrate digital tools into their workflow. For example, a recent graduate might use ChatGPT to help write code for a program or summarize documentation, viewing it simply as another tool at their disposal.
These challenges are not insurmountable, but they must be recognized and addressed by companies. A company that introduces AI tools in the workplace must take this generational gap into account to avoid creating divisions between enthusiastic early adopters and reluctant skeptics. It is important to accompany technological implementation with targeted training initiatives: practical workshops, concrete examples, peer mentoring (for instance, pairing a young “digital champion” with a senior colleague on an AI project). Additionally, it should be clearly communicated that AI is not meant to replace human experience but to enhance and accelerate it.
Another cultural aspect concerns the openness to change. Generations that have already witnessed several waves of innovation (from fax machines to PCs, from the internet to smartphones) may feel “tired” of constantly updating skills and procedures. It is essential to involve them by explaining the tangible benefits of AI: less repetitive work, tools that can simplify daily tasks, opportunities to update their role with more stimulating tasks that create efficiency. Sharing success stories of colleagues or peers who have adopted AI with satisfaction can help reduce resistance, allowing AI to become a shared asset rather than a source of division.
In summary, AI challenges both corporate and social cultures: it forces generations with different visions and skills to engage in dialogue. But if managed well, this confrontation can enrich everyone. Young people can learn from seniors the critical thinking and experience necessary to use AI in a truly useful and responsible way; seniors can learn from young people the familiarity with digital tools and the open-mindedness that allows them to embrace new things without fear. An inclusive approach, where everyone feels part of the transformation journey, will help businesses and society make the most of artificial intelligence.
Impact on business processes and productivity
What is the actual impact of artificial intelligence on business processes? In recent years, companies have begun integrating AI into a variety of functions and processes, yielding significant results in terms of productivity and operational efficiency. AI operates on multiple fronts: automating repetitive tasks, advanced data analysis to support decision-making, personalizing products/services on a large scale, and improving the speed and quality of task execution.
One major area of impact is process automation. Robotic Process Automation (RPA) systems enhanced with AI can perform routine administrative tasks—such as data entry, information extraction from documents, and form verification—much faster and without errors compared to a human. This frees up time for employees, allowing them to focus on more engaging and higher-value tasks. For example, in the financial sector, AI can automate invoice registration or cross-checking accounting transactions; in customer service, a virtual assistant can handle basic customer inquiries 24/7. These chatbots or automated response systems can resolve common issues (password resets, shipment tracking, commercial FAQs) without human intervention, reducing customer wait times and lowering management costs.
A often underestimated but essential element for AI to be beneficial is the quality of business data. This refers to machine-readable data, i.e., structured and organized data in formats that machines can easily read and interpret. If data is scattered across paper documents, isolated tables, or outdated formats, AI will struggle to be effective. Many companies are therefore investing in the digitization and standardization of data: centralizing information in well-designed databases, cleaning datasets of errors, and ensuring that different sources (CRM, ERP, spreadsheets) communicate with each other. This “behind-the-scenes” work is crucial for an AI algorithm to analyze and use the data profitably. The phrase "garbage in, garbage out" is often used: if AI is fed with poor-quality data, it will provide poor results. On the other hand, a data-driven company with reliable information can use AI to gain high-value insights and make better decisions.
The tangible effects of these applications can be seen in various indicators: reduced cycle times, fewer errors, lower operational costs, and often improved service or product quality. A study conducted by economists from Stanford and MIT, for example, found that call center operators increase their productivity by an average of 14% when using a conversational AI assistant; the increase rises to 35% for less experienced operators, who are able to close their skill gaps more quickly with the help of AI (The Impact of Artificial Intelligence on Productivity: Analysis of Available Research - Il Sole24Ore). This shows how AI can also act as an equalizer: helping those with less experience perform almost as well as experts by providing real-time suggestions and information during the work process. Looking to the future potential, according to a McKinsey analysis, up to 50% of call center activities could be automated thanks to AI, with a productivity increase estimated between 30% and 45%, while also improving the customer experience.
A look to the future: the next years of AI
Looking ahead to the coming years, we can expect artificial intelligence to become even more pervasive and powerful, but also to bring new challenges in technological, energy, geopolitical, and governance areas.
From a technological standpoint, we will likely witness the development of increasingly generalist and multimodal AI agents. This will pave the way for currently unthinkable or too expensive solutions, further democratizing access to AI.
Alongside these positive developments, however, lies the energy challenge. AI models, especially large ones, require immense amounts of energy for both initial training and continuous operation. As AI usage multiplies across sectors, the demand for energy in data centers will grow exponentially. In the coming years, making AI sustainable will become crucial: this means developing more efficient algorithms (able to achieve the same results with fewer calculations), hardware designed for lower energy consumption (specialized chips like the new GPUs and TPUs, or possibly the first hints of quantum computing for AI), and, above all, powering data centers with renewable energy. Already today, tech giants are investing in solar and wind farms to offset the carbon footprint of their servers.
On the geopolitical front, AI is already a field of global competition, and this will intensify. The next 5-6 years will see an acceleration of the so-called "AI race" between major powers. The United States and China are at the forefront: on one side, American companies (OpenAI/Microsoft, Google, Meta, Amazon...) dominate the development of the most advanced models and cloud platforms; on the other side, China is investing heavily in AI research, STEM education, and tech startups, with the declared goal of becoming the world leader by 2030. Europe, for its part, despite not having comparable giants in terms of proprietary models, is moving forward with investments and regulations.
The AI of 2030 will be (barring surprises) even more powerful, integrated into our lives, and likely so widespread that it will be perceived as invisible, part of the daily fabric - much like we take the internet or electricity for granted today.
It is up to us to ensure that this “smart electricity” illuminates the future without blinding us.